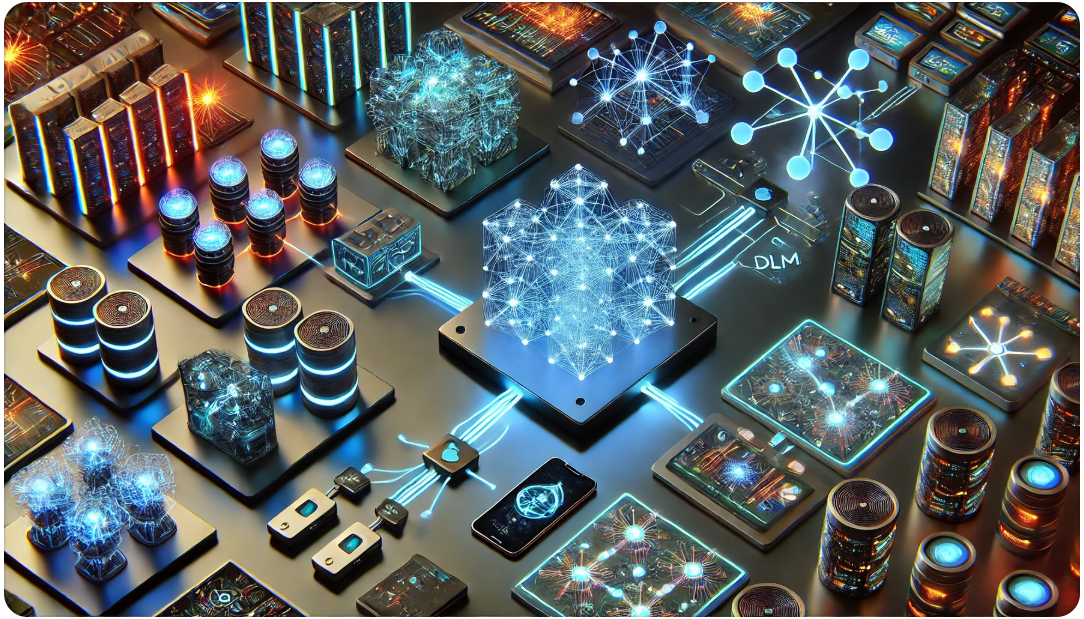
1. Technical Background and Definition
1.1 Definition and Characteristics of Small Language Models (SLMs) and Distributed Language Models (DLMs)
Small Language Models (SLMs)
Small Language Models (SLMs) are AI models with significantly fewer parameters than traditional large language models (LLMs). Typically, SLMs have parameter counts ranging from millions to a few billion, in contrast to LLMs, which can have hundreds of billions or even trillions of parameters. Key characteristics of SLMs include:
- Low computational requirements: SLMs require less memory and processing power, making them feasible for deployment on mobile devices, edge servers, and local machines.
- Faster inference: Due to their compact size, they can process inputs and generate responses more quickly than LLMs.
- Energy efficiency: Smaller models consume significantly less power compared to their larger counterparts.
- Privacy advantages: SLMs can run locally, ensuring data remains on the user’s device, thus enhancing security and privacy.
- Fine-tunability: SLMs are easier to fine-tune for specific tasks due to their reduced parameter count and computational cost.
Distributed Language Models (DLMs)
Distributed Language Models (DLMs) refer to language models that are either trained or deployed across multiple devices in a decentralized manner. This approach can take several forms:
- Federated Learning (FL): A method where multiple devices train a shared model collaboratively without sharing raw data. Instead, only model updates or gradients are exchanged.
- Peer-to-Peer (P2P) Training: A decentralized approach where model training occurs across a network of devices without a central coordinator.
- Distributed Inference: Large models are split into smaller components, which are then hosted across multiple machines or nodes, enabling efficient inference.
Key characteristics of DLMs include:
- Improved privacy: Since raw data remains on the device, it is less susceptible to breaches.
- Efficient resource utilization: DLMs leverage the collective computational power of multiple devices, reducing the need for powerful centralized servers.
- Resilience and fault tolerance: Training and inference are not dependent on a single machine, making the system more robust.
1.2 Comparison with Large Language Models (LLMs)
Feature | Small Language Models (SLMs) | Large Language Models (LLMs) | Distributed Language Models (DLMs) |
---|---|---|---|
Size | Typically 1M-10B parameters | 100B+ parameters | Varies depending on distribution |
Computational Cost | Low | Extremely high | Distributed across nodes |
Inference Speed | Fast | Slower due to size | Dependent on network and setup |
Training Approach | Focused on efficiency | Requires massive datasets and compute | Federated or collaborative training |
Deployment | Can run on mobile devices, edge servers | Requires powerful GPUs/TPUs | Can operate across multiple devices |
Privacy | Higher (local processing) | Lower (cloud-based) | High (data remains local in FL) |
1.3 Representative Examples of SLMs and DLMs
- SLMs:
- DistilBERT (Hugging Face): A distilled version of BERT that is 40% smaller while retaining 97% of performance.
- Phi-3 (Microsoft): A high-performance small model optimized for efficiency and long-context understanding.
- LLaMA 3 (Meta, upcoming): Smaller variants optimized for edge deployment.
- Mistral 7B: A competitive open-source SLM performing well on various benchmarks.
- TinySwallow-1.5B (Sakana AI): A Japanese language model optimized for edge computing.
- DLMs:
- Petals: A P2P system where users collaboratively serve LLMs over a network.
- Flower (Federated Learning Framework): A toolkit enabling decentralized training of language models.
- Google’s Federated Learning AI: Used for GBoard and other personalized AI applications.
2. Development Trends and Major Players
2.1 Recent Research and Development Trends
- Increasing efficiency of SLMs through architectural optimizations and better training techniques.
- Advances in knowledge distillation and model pruning to make small models more effective.
- Federated and decentralized training becoming a major focus due to privacy concerns and compute distribution.
2.2 Efforts of Major Companies and Research Institutes
Organization | Key Contributions to SLMs/DLMs |
Gemma, Federated AI for mobile devices | |
Meta | LLaMA open-source models, ongoing SLM research |
OpenAI | GPT-4o Mini, model compression research |
Microsoft | Phi-3, Federated AI research |
Hugging Face | Petals, DistilBERT, federated learning initiatives |
Stability AI | StableLM series, lightweight models |
Sakana AI | TinySwallow-1.5B, AI constellation approach for distributed intelligence |
3. Implementation and Challenges of Distributed Language Models
3.1 Methods of Distributed Learning and Inference
- Federated Learning (FL): Enables decentralized training without exposing raw data.
- P2P Distributed AI: Eliminates the need for central coordination.
- Distributed Model Serving: Splitting LLMs across multiple devices for efficient inference.
3.2 Challenges
Challenge | Description |
Communication Overhead | High data exchange in FL can slow training |
Security Risks | Model updates may be manipulated (poisoning attacks) |
Hardware Constraints | Edge devices have limited compute and memory |
4. Future Technology Trends and Market Outlook
4.1 Predictions for SLM/DLM Evolution
- Hybrid AI Ecosystems: Integration of cloud-based LLMs with on-device SLMs.
- AI Personalization: Edge AI models will adapt to individual users.
- Energy-Efficient AI: Adoption of low-power AI chips for local inference.
4.2 Use Cases
- Edge AI: AI-powered smartphones and IoT devices.
- Healthcare AI: Federated models for medical applications.
- Enterprise AI: Secure AI deployed on-premises.
5. Security Risks, Ethical Issues, and Countermeasures
5.1 Security Concerns
- Model Poisoning: Malicious updates can corrupt models.
- Privacy Attacks: Federated updates may leak sensitive data.
- Model Theft: Unauthorized extraction of proprietary models.
5.2 Ethical Considerations
- Bias in Small Models: Training on narrow datasets may introduce biases.
- Lack of Oversight: Decentralized models pose challenges for accountability.
5.3 Countermeasures
- Secure Aggregation: Encrypt model updates in federated learning.
- Bias Audits: Systematic testing of model fairness.
- Governance Frameworks: Implementing AI safety regulations.
Conclusion
SLMs and DLMs represent the next frontier of AI development. With advances in efficiency, federated learning, and distributed inference, AI will become more accessible, privacy-preserving, and cost-effective. However, challenges in security, bias, and governance must be addressed to ensure responsible deployment.